Hidden Pitfall: Setting Customer Expectations
Published on 17 June 2022 by Jeffrey Wan
Can you name some of the challenges faced by AI start-ups? Sourcing capital and funding, identifying niche markets, positioning against traditional industry leaders or business models… Indeed, the above (among over thousands of other obstacles you may think of) are rather common for start-ups, yet when it comes to start-ups that build AI, there is one thing that particularly applies, matters a lot, and is often overlooked—setting customer expectations.
AI start-ups are like many other tech start-ups, but still different. What’s unique about AI building is the highly experiment-driven nature of machine learning. While the tradition software development process is established based on a prescribed specification and deliver an end-product according to the outline, machine learning relies heavily on the data size and as a result outcome can hardly be accurately predicted beforehand. Even if it’s perfect at initial deployment, its performance may go downhill over time due to reasons that are unexpected and hard to identify, because it’s the machine that learns the habits and makes the mistakes, which is absolutely out of human’s plan!
The most prominent example of setting wrong expectations would be the timeline for launching auto-pilot driving technology, which many people expected to be ready really quickly and that everyone can soon sleep-drive without any steering wheel or dashboard. Development does take time. Where the industry has put a foot wrong is that the hype and presentation led to awfully wrong expectation. The public was simply over-expecting.
In DeepTranslate, there is one thing you can expect—we only promise what we can deliver. We once had a potential customer saying that our machine translation isn’t perfect, which we know it isn’t perfect—as our AI isn’t designed to completely replace human translators, but instead aim at streamlining the workflow to help businesses reduce costs and time. Fortunately, our AI developers and translation team work together to screen data of good quality and train our AI and review results from time to time, which together with feedback and additional data from clients, a greater database can usually improve training results.
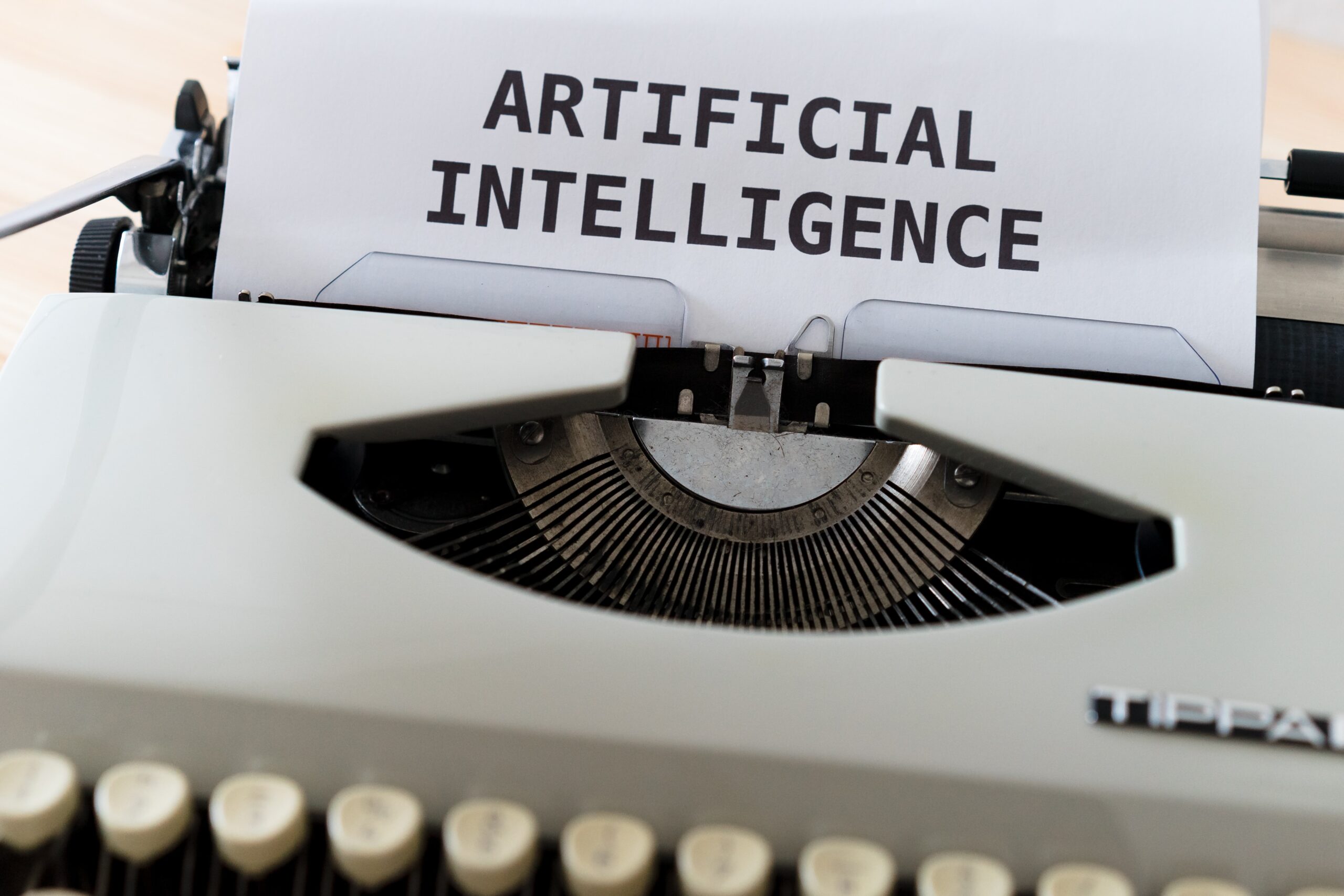